Condition Based Maintenance
Condition-based maintenance (CBM) is a data-driven approach to maintenance that relies on real-time data from sensors like vibration sensors and accelerometers to monitor the health of assets and perform maintenance only when necessary.
Here’s a step-by-step explanation of how to use this data to predict failures, monitor assets, and reduce maintenance costs:
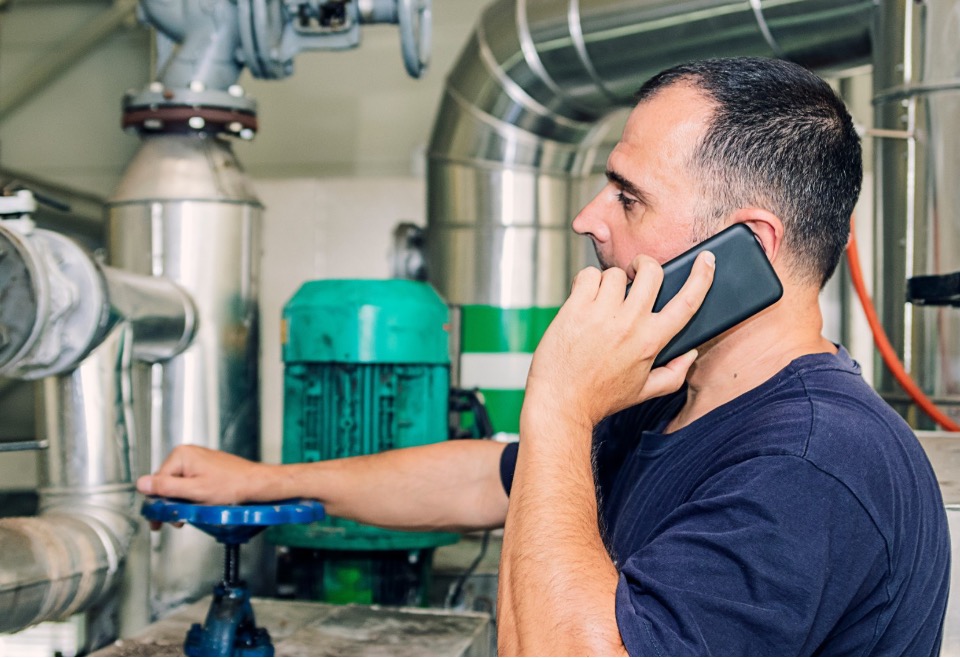
Data-Driven Approach
The AVEVA PI System is a data infrastructure platform that can be used to collect, store, and analyze time-series data from various sources, including these sensors.Here’s a step-by-step explanation of how to use this data to predict failures, monitor assets, and reduce maintenance costs:
Data Collection
Installed vibration sensors and accelerometers on the assets to monitor are the key components. These sensors collect real-time data, including vibrations, temperature, and other relevant parameters. Connected sensors to a DCS/BAS/SCADA or controller that can process and transmit the data to the AVEVA PI server.
Data Ingestion
Set up data connectors or interfaces within AVEVA PI to ingest the time-series data from the sensors. This can be done using various BACNet/Modbus protocols and communication standards. We can use Data Diodes to provide data security to the device for Cyber protocol measures.
Data Storage
AVEVA PI server stores the incoming time-series data, allowing for historical analysis and trend tracking. The data is organized into a structured database, making it easily accessible for analysis. DSA has an AWS Cloud option to allow safe storage and real time access to the Data.
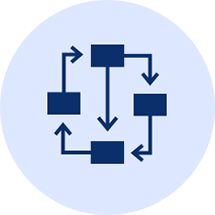
“The rise in cost for time-based maintenance has triggered more opportunities to use data to predict failures in assets. With the WatchPost Framework, we can utilize sensor data in real time and use predictive algorithms that reduce the cost of maintenance while keeping assets online and reliable.”
Asset Framework Development
We develop an asset framework within the AVEVA PI server to represent the assets you are monitoring with ASHRAE 223 endorsed Haystack Schema. This framework helps organize the data by asset type, location, and criticality, making it easier to manage and analyze.
Data Preprocessing
We use data preprocessing techniques to clean and filter the sensor data. This can involve removing outliers, aggregating data, and aligning it with asset-specific parameters.
Predictive Analytics
We employ predictive analytics and machine learning algorithms to analyze sensor data. These algorithms can identify patterns, anomalies, and early indicators of asset degradation or failure.
Thresholds and Alarms
We set up threshold values and alarms within the AVEVA PI server. When the sensor data exceeds predefined thresholds, the system generates alerts, notifying maintenance teams of potential issues.
Cost Savings and Efficiency
By performing maintenance only when needed, you can reduce operational and maintenance costs, extend the lifespan of assets, and improve overall efficiency.
Proactive Maintenance
With real-time data and predictive insights, maintenance teams can proactively schedule maintenance activities only when the data indicates that maintenance is required. This approach minimizes unplanned downtime and reduces unnecessary maintenance costs.
Reporting and Decision Support
AVEVA PI provides tools for generating reports, dashboards, and visualization of asset health and performance. Key stakeholders can access this information to make data-driven decisions regarding maintenance and asset management.